The Cloud AI Market was valued at USD 5.2 billion in 2020 and is expected to reach USD 13.1 billion by 2026, registering a CAGR of 20.3%, during the period of 2021-2026. (Source: Mordor Intelligence)
Analytics and machine learning (ML) are currently among the fastest-growing workloads. Businesses seek to exploit the insights generated for decision support, customer engagement, cost reduction, risk management, and other purposes.
Unfortunately, firms seeking to harness the potential of analytics and machine learning confront major difficulties. Many organizations lack people with analytics and machine learning knowledge or training. Most lack the necessary infrastructure for it. They cannot store the data required to create and train models and the computational capability to operate the models and workloads.
Making matters more complex, there are several phases to implementing analytics and machine learning initiatives. Among them are building/training, tuning/testing, and deploying/running in production. Each level has its own set of computation and storage needs. For example, the building/training stage typically requires large datasets, the tuning/testing stage necessitates many high-performance computing cores or instances, and the deploying/running stage necessitates dependable computing power that delivers results or insights promptly that businesses can act on.
And those requirements may change dramatically over time. For example, a financial services organization may desire to improve an analytics or machine learning-based model or application by adopting a fresh economic dataset and retraining it. Alternatively, a corporation may make a production version of an analytics and machine learning model, application, or tool available to a restricted group of users. The organization will then be able to use it across the entire organization.
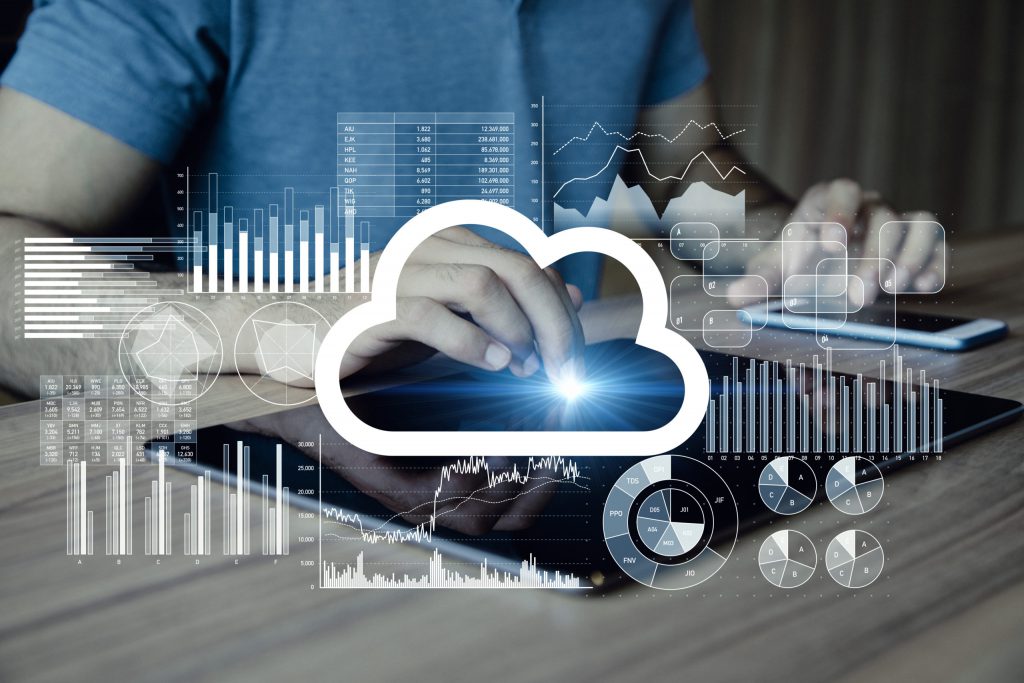
Hybrid cloud steps in
Major public cloud providers want developers and data scientists to build, test, and deploy machine learning models on their clouds. Each service provides a variety of data storage choices, such as serverless databases, data warehouses, data lakes, and NoSQL datastores.
93 percent of enterprises have a multi-cloud strategy; 87 percent have a hybrid cloud strategy. (Source: G2)
They provide well-known machine learning frameworks such as TensorFlow and PyTorch. All offer expanding capabilities to serve the whole analytics and machine learning life cycle.
Despite these possibilities, most firms prefer to maintain certain portions of their analytics and machine learning initiatives on-premises. For example, a company may rely on a big customer dataset stored on an outdated system. Migrating such a dataset to the public cloud would be impractical (and certainly quite costly). The prudent course of action would be to leave the data alone.
Enterprises saw an estimated growth rate of 17 percent after adopting Hybrid cloud. (Source: Accenture)
That is where the hybrid cloud comes in. To maximize a single workload, a hybrid cloud may operate several cloud infrastructures in an interoperable manner. How can this assist with analytics and machine learning? A hybrid cloud strategy allows firms to pick and choose which components of their analytics and machine learning processes to maintain on-premises and which to employ in the cloud. For example, a company may rely on cloud storage to accommodate the massive data required to train models. Alternatively, companies may leverage analytics and machine learning-specific instances to speed up workloads.
Each cloud service (data storage, compute power, analytics and ML acceleration via specific processors, and so on) provides the ability to scale up or down to meet the elastic demands of analytics and ML workloads.
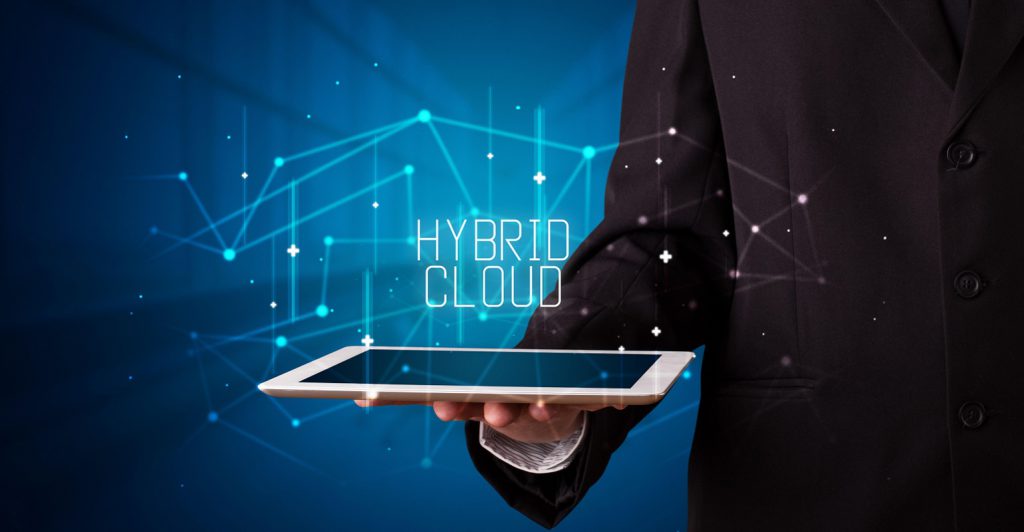
Well-suited to analytics and ML
A hybrid cloud has several advantages for analytics and machine learning, and it is well-suited to address changing demands over time. On the other hand, hybrid cloud environments can be quite complicated. And this can cause issues.
There are several challenges at hand. Many tools for managing and troubleshooting infrastructure or workload performance are built for a single environment. A company may be aware of what is going on on its grounds. However, this does not apply to the public cloud. Furthermore, while cloud providers may supply their tools and management systems, most will not work with on-premises workloads or other cloud services.
This is a concern because, when designing, testing, and deploying analytics and ML on a hybrid platform, these different technologies make it challenging to debug difficulties, uncover root cause issues, discover issues in the making, or notice abnormalities that are precursors to security and performance problems.
Firms need a suitable solution that would assist them in creating, managing, governing, and optimizing a complex hybrid cloud infrastructure. It should also integrate the administration of various public clouds’ physical resources with a private cloud in an on-premises data center. Those are the fundamentals.