Introduction: Revolutionizing the Landscape
The field of generative AI hardware is rapidly evolving, with new technologies emerging that are expected to revolutionize the industry. Two of the most promising technologies are quantum computing and neuromorphic chips. In this article, we will explore the potential of these technologies and their impact on the future of generative AI hardware.
Quantum Computing: A Quantum Leap into the Future
Quantum computing is a technology that uses quantum-mechanical phenomena to perform operations on data. It is expected to be a game-changer in the field of generative AI hardware. According to a report by Research and Markets, the quantum computing market is expected to grow rapidly over the next decade and reach approximately $1.78 billion by 2025. This growth is expected to be driven by the increasing demand for quantum computing in various industries, including healthcare, finance, and transportation.
Advantages of Quantum Computing
Quantum computing has several advantages over traditional computing hardware. For example, it can perform complex calculations much faster than traditional computers. This makes it ideal for use in AI and machine learning applications that require large amounts of processing power. Additionally, quantum computing is highly scalable, which means that it can be used to process large amounts of data quickly and efficiently.
Challenges of Quantum Computing
Despite its many advantages, quantum computing also has several challenges that need to be addressed. One of the biggest challenges is the need for specialized hardware to support quantum computing operations. This hardware is expensive and difficult to manufacture, which makes it difficult for smaller companies to enter the market. Additionally, quantum computing requires significant investment in research and development to optimize its performance.
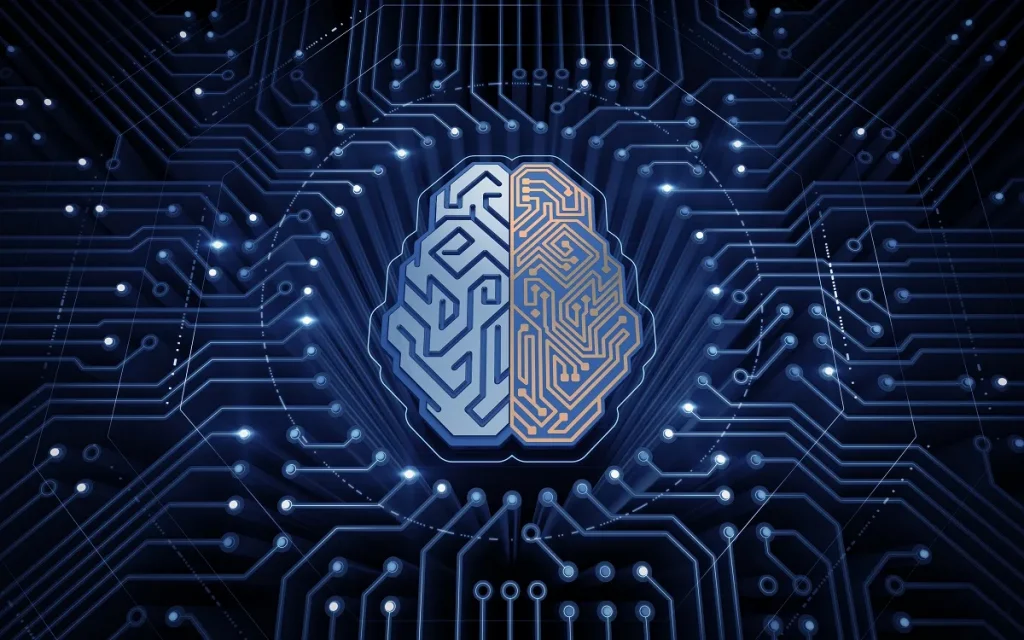
Neuromorphic Chips: Mirroring the Mind
Neuromorphic chips are designed to mimic the structure and function of the human brain. They are expected to be highly efficient in processing large amounts of data and are ideal for use in AI and machine learning applications. According to a report by Sandia researchers, neuromorphic chips are significantly more energy-efficient than conventional computing hardware. This makes them an attractive option for use in AI and machine learning applications that require large amounts of processing power.
Advantages of Neuromorphic Chips
Neuromorphic chips have several advantages over traditional computing hardware. For example, they are highly efficient in processing large amounts of data and are ideal for use in AI and machine learning applications. Additionally, they are significantly more energy-efficient than traditional computing hardware, which makes them an attractive option for use in applications that require large amounts of processing power.
Challenges of Neuromorphic Chips
Despite their many advantages, neuromorphic chips also have several challenges that need to be addressed. One of the biggest challenges is the need for specialized hardware to support neuromorphic computing operations. This hardware is expensive and difficult to manufacture, which makes it difficult for smaller companies to enter the market. Additionally, neuromorphic computing requires significant investment in research and development to optimize its performance.
The Future of Generative AI Hardware
The combination of quantum computing and neuromorphic chips is expected to bring about a new era of generative AI hardware. According to McKinsey research, generative AI features stand to add up to $4.4 trillion to the global economy annually. This is a significant increase from the current value of the generative AI market, which is estimated to be around $1.4 trillion.
Charting the Course: The Future of Generative AI Hardware
The potential of generative AI hardware is enormous. It has the potential to transform industries and create new opportunities for businesses. For example, generative AI can be used to create personalized content for customers, such as customized product recommendations and personalized marketing messages. It can also be used to optimize supply chain management and improve the efficiency of manufacturing processes.
Challenges of Generative AI Hardware
However, there are also challenges associated with the development of generative AI hardware. One of the biggest challenges is the need for large amounts of data to train the AI models. This requires significant investment in data collection and storage infrastructure. Another challenge is the need for specialized hardware to support the processing requirements of generative AI models.
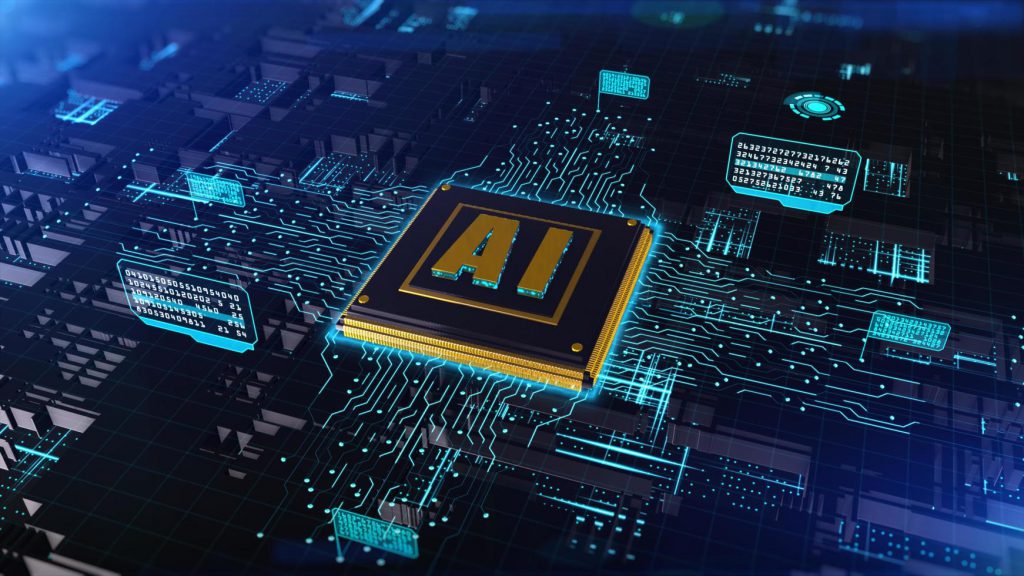
Conclusion
The future of generative AI hardware is promising. Quantum computing and neuromorphic chips are expected to be the key drivers of growth in the industry. The potential of generative AI is enormous, and it has the potential to transform industries and create new opportunities for businesses. However, there are also challenges associated with the development of generative AI hardware. Despite these challenges, the future of generative AI hardware looks bright, and it is expected to bring about a new era of AI and machine learning.