Floods are among the most devastating natural disasters, causing significant loss of life and property. Traditional flood forecasting methods often fall short in providing timely and accurate predictions, leading to inadequate disaster response. However, the advent of exascale computing models is revolutionizing this field, offering unprecedented accuracy and a crucial 24-hour lead time in flood forecasting. This article delves into how these models are transforming flood forecasting and disaster response, highlighting their impact on stakeholders.
The Power of Exascale Computing
Exascale computing refers to systems capable of performing at least one exaflop, or a billion billion (10^18) calculations per second. This immense computational power enables the processing of vast amounts of data at unprecedented speeds, making it possible to create highly detailed and accurate flood models. These models integrate various data sources, including satellite imagery, weather forecasts, and hydrological data, to predict flood events with remarkable precision.
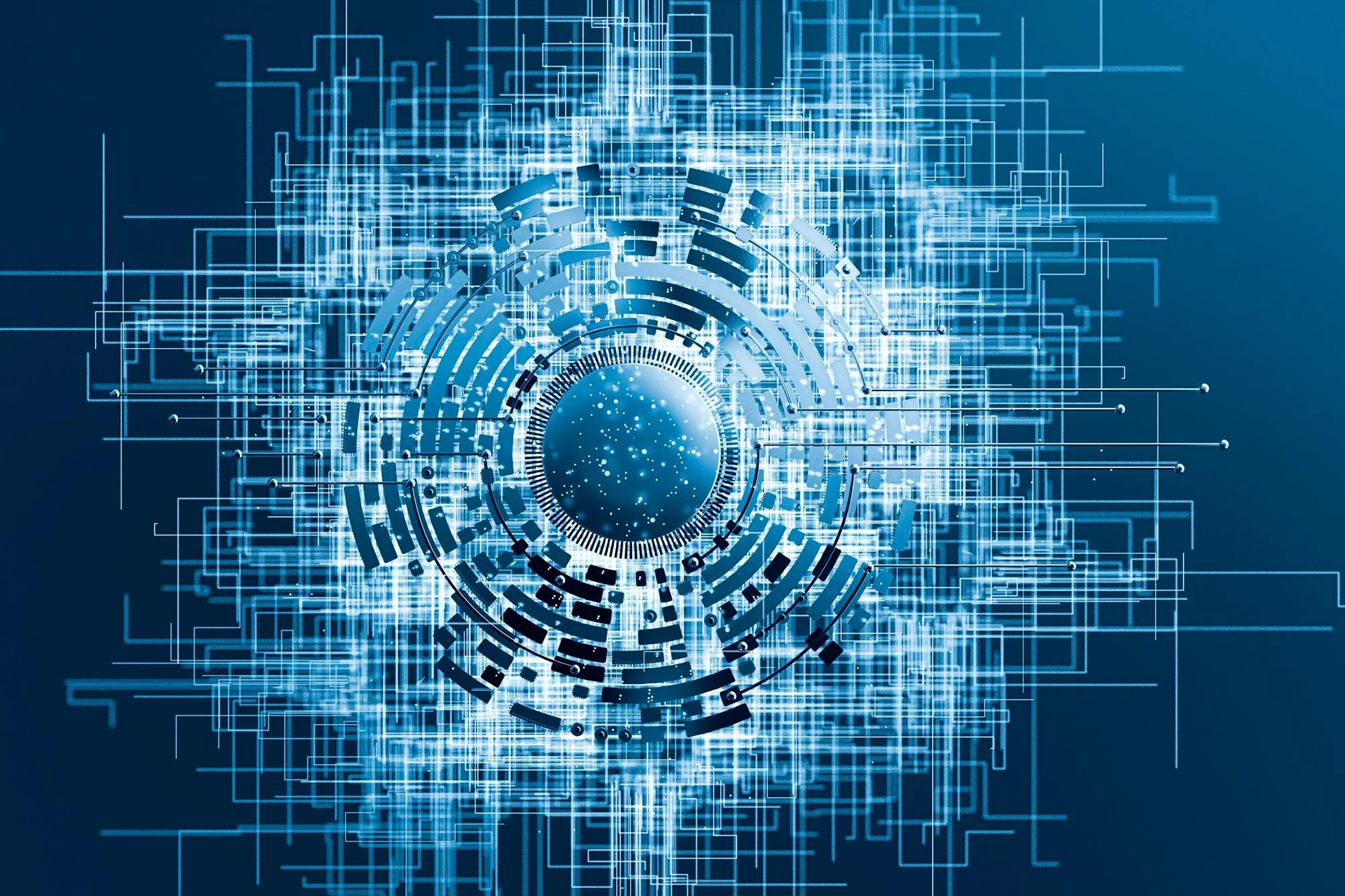
Enhanced Data Integration
Exascale models can process and integrate data from multiple sources in real-time. For instance, satellite data provides high-resolution images of water bodies, while weather forecasts offer insights into precipitation patterns. By combining these data sets, exascale models can simulate flood scenarios with high accuracy. This integration is crucial for stakeholders, as it allows for more informed decision-making and resource allocation.
24-Hour Lead Time: A Game Changer
One of the most significant advancements brought by exascale models is the ability to provide a 24-hour lead time in flood forecasting. This extended lead time is critical for effective disaster response and mitigation efforts.
Early Warning Systems
With a 24-hour lead time, early warning systems can be activated well in advance of a flood event. This allows authorities to issue timely alerts to affected communities, enabling them to evacuate and take necessary precautions. According to recent studies, early warnings can reduce flood-related fatalities by up to 30% (Source: Google Research Blog).
Resource Allocation
A longer lead time also facilitates better resource allocation. Emergency services can be strategically deployed to high-risk areas, ensuring that rescue operations are swift and efficient. Additionally, critical infrastructure, such as hospitals and power plants, can be fortified against potential flood damage. This proactive approach significantly reduces the economic impact of floods, which is estimated to be around $10 billion annually (Source: Hydrology and Earth System Sciences).
Improved Accuracy and Reliability
Exascale models not only extend lead times but also enhance the accuracy and reliability of flood forecasts. Traditional models often struggle with predicting the precise location and intensity of floods. In contrast, exascale models leverage advanced algorithms and machine learning techniques to deliver highly accurate predictions.
Case Study: The 2023 Monsoon Season
During the 2023 monsoon season, exascale models were deployed in flood-prone regions of India and Bangladesh. The models achieved an R² score of over 99%, accurately predicting water levels within a 15 cm error margin more than 90% of the time (Source: Nature). This level of precision was instrumental in mitigating the impact of floods on millions of people.
Stakeholder Confidence
The improved accuracy of exascale models boosts stakeholder confidence in flood forecasts. Governments, disaster management agencies, and insurance companies can rely on these predictions to make informed decisions. For instance, insurance companies can better assess risk and set premiums accordingly, while governments can allocate funds for flood prevention and relief efforts more effectively.
Challenges and Future Directions
Despite their numerous advantages, exascale models face certain challenges. The primary challenge is the need for substantial computational resources and infrastructure. Developing and maintaining exascale systems requires significant investment, which may be a barrier for some regions.
Addressing Computational Demands
To address these challenges, collaborative efforts between governments, private sector, and international organizations are essential. Investments in cloud computing and shared infrastructure can help make exascale models more accessible. Additionally, ongoing research and development are crucial for optimizing these models and reducing their computational demands.
Expanding Coverage
Future efforts should focus on expanding the geographical coverage of exascale models. Currently, these models are primarily deployed in regions with high flood risk. However, expanding their reach to other vulnerable areas can further enhance global flood resilience. This expansion requires continuous data collection and model refinement to ensure accuracy across diverse landscapes.
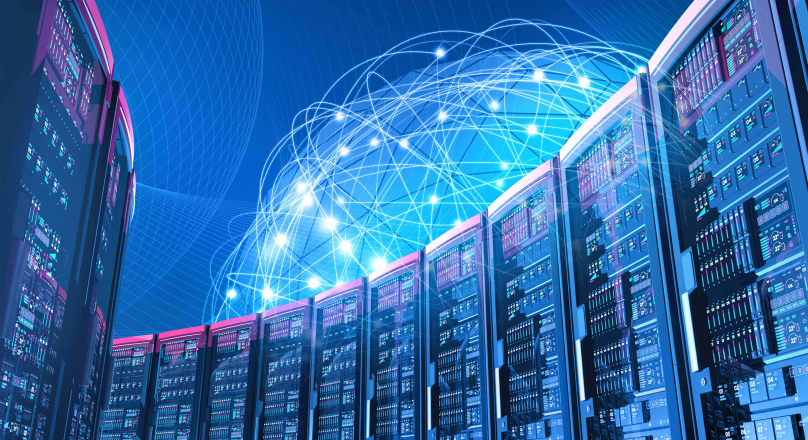
Conclusion
Exascale computing models represent a transformative advancement in flood forecasting and disaster response. By providing a 24-hour lead time and unparalleled accuracy, these models empower stakeholders to make informed decisions, allocate resources effectively, and ultimately save lives. While challenges remain, the potential benefits of exascale models far outweigh the obstacles. As technology continues to evolve, exascale models will play an increasingly vital role in building resilient communities and mitigating the impact of floods worldwide.