Introduction
The integration of Artificial Intelligence (AI) and Machine Learning (ML) into business processes has the potential to revolutionize small and medium-sized businesses (SMBs), providing them the competitive edge once reserved for larger enterprises with substantial resources. One of the most transformative aspects of AI and ML technology is MLOps (Machine Learning Operations), which combines ML model development and operations to automate and streamline workflows. This article explores how MLOps can empower small businesses, highlighting its impact on efficiency, growth, and innovation.
Understanding MLOps
MLOps refers to a set of practices that aim to deploy and maintain machine learning models in production reliably and efficiently. By integrating development (Dev) and operations (Ops) practices with machine learning (ML), MLOps facilitates automated management of the machine learning lifecycle, from data collection and preprocessing to model deployment and monitoring. MLOps ensures the operational stability of ML models and enhances their continuous improvement and scalability.
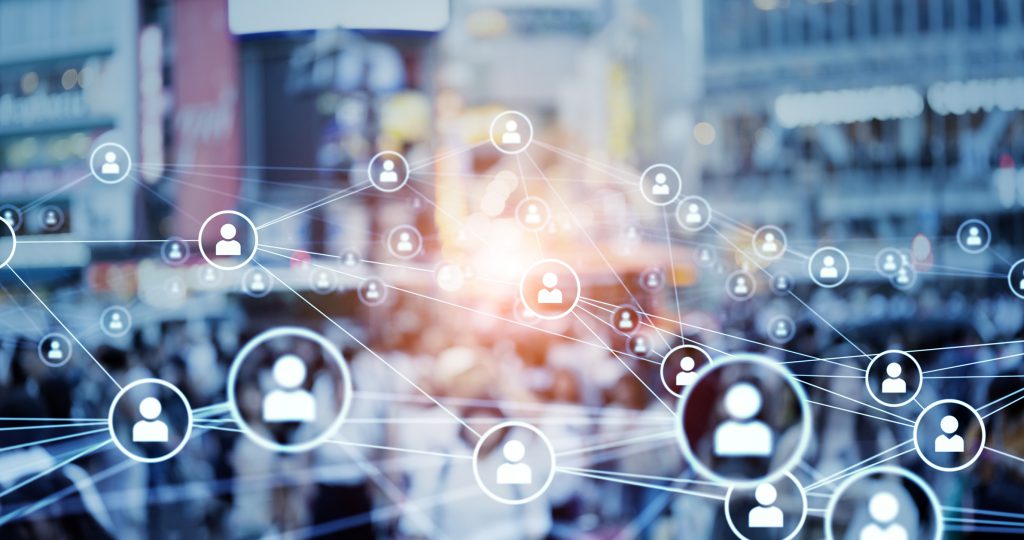
Enhancing Operational Efficiency
Automating Routine Tasks
One of the primary benefits of MLOps for small businesses is its ability to automate repetitive and time-consuming tasks. Utilizing AI and ML can automate data entry, email filtering, scheduling, and other routine operations. This automation reduces human errors and increases both efficiency and productivity. For example, generative AI can draft marketing copy, social media posts, and blog articles, allowing content creation at scale with fewer resources.
Streamlining Workflows
MLOps orchestrate workflows by managing the machine learning pipeline, from data preprocessing to model deployment and monitoring. This comprehensive approach ensures reproducibility, versioning, and collaboration, enabling businesses to streamline their processes efficiently. By adopting MLOps, companies can free up their teams to focus on strategic and innovative tasks rather than mundane operations.
Driving Business Growth
Data-Driven Decision Making
MLOps empowers small businesses to harness the full potential of their data. By deploying ML models that provide insights into customer behavior, market trends, and operational efficiencies, companies can make data-driven decisions crucial for growth. For instance, data analysis platforms can offer valuable insights into customer preferences, helping businesses tailor their offerings accordingly.
Enhancing Customer Experience
AI and ML models can significantly enhance customer service by automating responses to frequently asked questions and personalizing customer interactions. Chatbots can handle routine inquiries, allowing human agents to address more complex issues, improving customer experience. Additionally, predictive models can anticipate customer needs, enabling proactive service delivery.
Overcoming Challenges with MLOps
Addressing Resource Constraints
Small businesses often face constraints in terms of financial resources and technical expertise. Implementing MLOps, however, can be made more accessible through cost-efficient solutions like open-source platforms. Additionally, seeking expert advice for the initial setup or partnering with AI development companies can help SMBs overcome these barriers without incurring excessive costs.
Ensuring Data Quality and Security
Data quality and security are critical for successfully implementing AI and ML models. MLOps emphasizes the importance of continuous monitoring and data management to ensure the accuracy and reliability of the models. Implementing robust data governance frameworks can help businesses manage data quality while adhering to privacy and security regulations.
Best Practices for Implementing MLOps
Comprehensive Discovery Phase
Initiate a thorough discovery phase to identify the most critical business problems that AI can address. This foundational step ensures that the AI infrastructure aligns with business priorities and capabilities.
Reference Architecture for MLOps
Develop a clear reference architecture that supports AI initiatives and guides the selection of appropriate technologies. This architecture is crucial for the efficient operationalization of AI solutions.
Continuous Learning and Development
Foster a culture of continuous learning to keep pace with the latest advancements in AI and ML. Providing ongoing training and development for staff ensures that the business remains competitive and innovative.
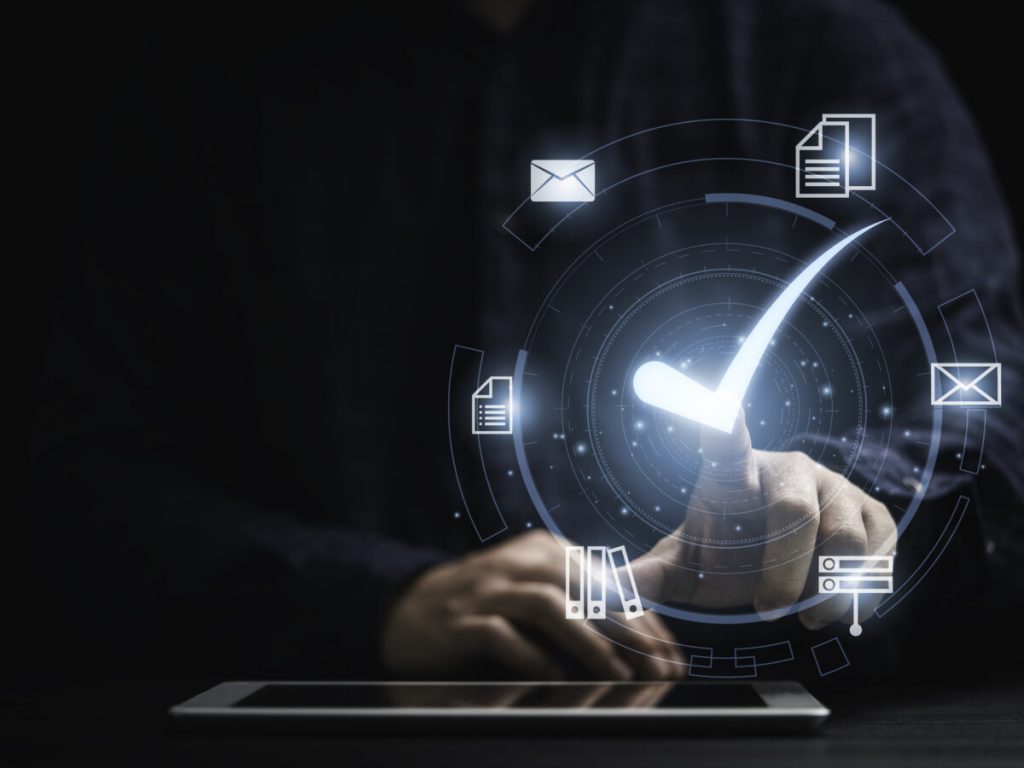
Conclusion
MLOps holds immense potential to empower small businesses by enhancing efficiency, driving growth, and fostering innovation. Through the automation of routine tasks, streamlined workflows, and data-driven decision-making, MLOps enables enterprises to harness the power of AI effectively. Overcoming resource constraints and data quality challenges requires strategic planning, expert guidance, and a commitment to continuous learning. By adopting MLOps, small businesses can unlock new opportunities, achieve operational excellence, and sustain long-term growth in a competitive marketplace.