Definition of Precision Agriculture
Precision Agriculture, also known as Smart Farming or Precision Farming, is an approach that leverages technology to precisely manage agricultural resources, thereby enhancing crop productivity and sustainability. It involves the integration of various technologies like GPS, sensors, IoT devices, and data analytics to make informed decisions about crop cultivation.
Importance of Advancements in Farming
As the global population continues to grow, the demand for food is increasing exponentially. Advancing farming techniques is crucial to meet this demand while preserving natural resources and minimizing environmental impacts. Precision Agriculture, powered by deep learning infrastructure, offers a promising solution to address these challenges effectively.
Deep Learning in Agriculture
Understanding Deep Learning
Deep learning is a subset of artificial intelligence (AI) that imitates the way the human brain processes information. It involves training artificial neural networks on vast amounts of data to recognize patterns, make predictions, and perform complex tasks without explicit programming.
Applications of Deep Learning in Farming
In agriculture, deep learning algorithms can be applied to a wide range of tasks, including:
- Crop Monitoring: Using satellite and drone imagery, deep learning models can identify crop health, growth stages, and detect diseases or nutrient deficiencies.
- Weed and Pest Control: Deep learning can differentiate between crops and weeds, enabling precision spraying and minimizing the use of herbicides.
- Predictive Analytics: By analyzing historical data, deep learning models can predict crop yields, market demand, and optimal planting times.
Benefits of Advancing Precision Agriculture
Increased Efficiency
Precision Agriculture optimizes resource usage, such as water, fertilizers, and pesticides, by applying them only where and when needed. This targeted approach reduces waste and lowers production costs, leading to increased overall efficiency.
Reduced Resource Wastage
Conventional farming often involves blanket application of resources across entire fields, leading to inefficient use and wastage. Precision Agriculture, powered by deep learning insights, ensures that resources are utilized judiciously, minimizing environmental impact.
Higher Crop Yields
By employing data-driven decision-making, farmers can make informed choices about crop varieties, planting times, and cultivation practices. This leads to better yields and improved crop quality.
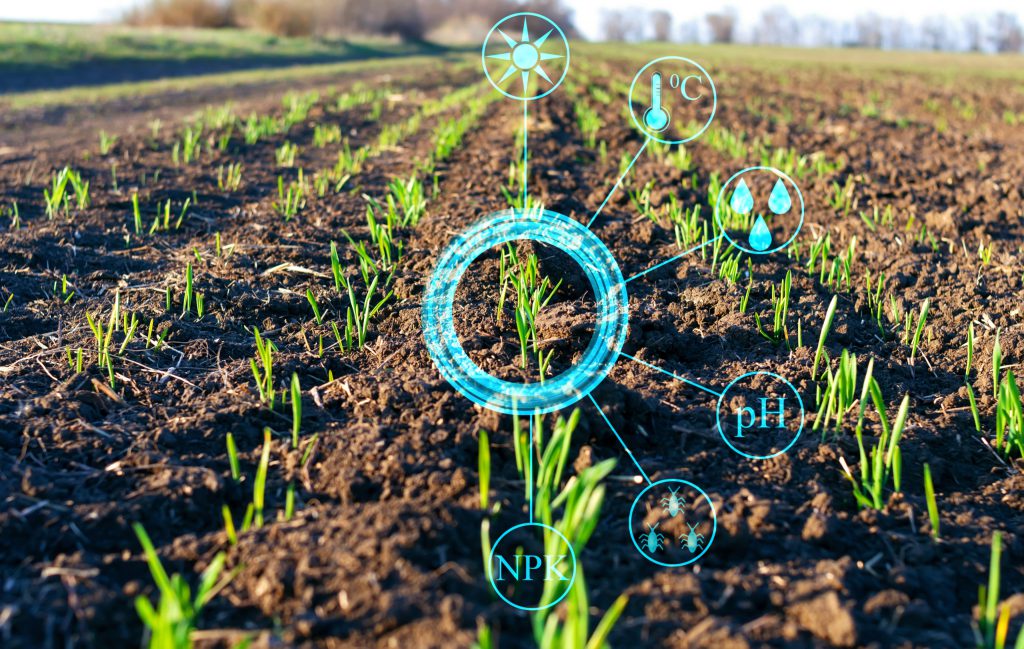
Components of Deep Learning Infrastructure in Farming
Sensors and IoT Devices
The foundation of Precision Agriculture lies in data collection, and this is facilitated by sensors and Internet of Things (IoT) devices. Soil moisture sensors, weather stations, and drones equipped with various sensors provide real-time data on environmental conditions and crop health.
Data Collection and Analysis
The data collected from sensors and other sources need to be processed and analyzed to derive valuable insights. Deep learning algorithms can efficiently analyze vast datasets, identifying patterns and trends that may not be apparent through traditional methods.
Automated Decision-Making
Deep learning models can automate decision-making processes, such as when and where to irrigate, apply fertilizers, or deploy pest control measures. This automation streamlines farming operations and ensures timely actions.
Challenges in Implementing Deep Learning in Agriculture
Data Privacy and Security
As farms become more data-driven, concerns about data privacy and security arise. Farmers must ensure that their data is protected from unauthorized access and that it is used responsibly by service providers.
High Initial Investment
Adopting Precision Agriculture and implementing deep learning infrastructure requires significant initial investment in technology, training, and equipment. This cost may be a barrier for small-scale farmers.
Adoption Barriers
Some farmers may be resistant to adopting new technologies due to a lack of awareness, technical know-how, or the perceived complexity of implementation. Effective training and support are essential to overcome these barriers.
The Future of Precision Agriculture
Integration of AI and Robotics
The future of Precision Agriculture lies in the seamless integration of AI with robotics. AI-powered robots and drones can perform tasks such as planting, harvesting, and crop monitoring with increased efficiency and precision.
Sustainable Farming Practices
As the world focuses on sustainability, Precision Agriculture will play a vital role in promoting eco-friendly farming practices. By using data-driven insights, farmers can adopt practices that minimize environmental impact while ensuring profitability.

Conclusion
Advancing Precision Agriculture through the integration of deep learning infrastructure represents a transformative shift in modern farming. The ability to make data-driven decisions, automate processes, and optimize resource usage offers immense potential for sustainable and efficient agricultural practices. Embracing these technological advancements will not only benefit farmers by increasing yields and reducing costs but also contribute to global food security and environmental preservation.