Introduction
The drug development landscape is evolving rapidly, propelled by groundbreaking technologies that shorten timelines and enhance predictive accuracies. Among these innovations, Graphics Processing Units (GPUs) have emerged as a transformative force, enabling the creation of more reliable predictive models. With the advent of GPU servers, researchers and pharmaceutical companies can now remarkably improve model accuracy. This article explores how GPU servers revolutionize drug development, underscoring their impact on accuracy and efficiency.
The Need for Predictive Models in Drug Development
Complex Biological Data
Drug development is an intricate process that requires the analysis of vast amounts of biological data. These datasets include genomic sequences, protein structures, and metabolic pathways. Processing and interpreting this complex information quickly and accurately is paramount to identifying viable drug candidates and minimizing costly late-stage failures.
Traditional Computational Limitations
Historically, Central Processing Unit (CPU)-based systems have been employed to develop predictive models in drug discovery. However, their sequential processing capabilities often fall short in handling the massive parallelism required to analyze biological data efficiently. This limitation leads to prolonged development cycles and less accurate predictive models, constraining the ability to swiftly bring new drugs to market.
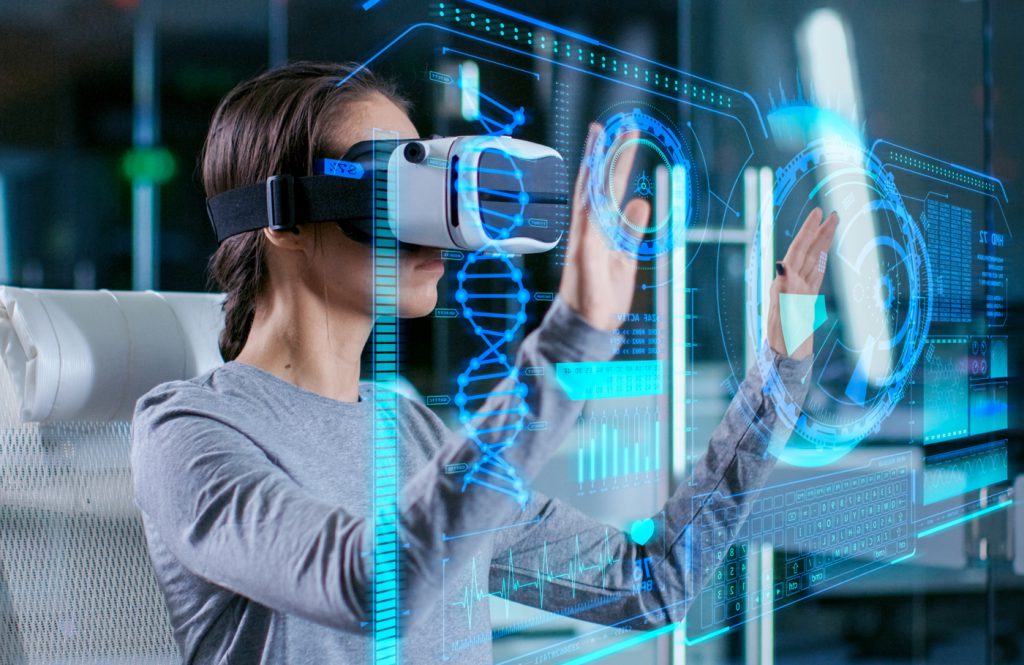
How GPU Servers Transform Drug Development
Understanding GPU Servers
GPUs are engineered for massively parallel processing, enabling simultaneous computations across thousands of cores. This capability makes them highly suited for tasks that involve extensive data processing and algorithmic computations, such as those required in drug development. GPU servers utilize multiple GPUs to work in collaboration, distribute the computational load, and significantly enhance processing speed and accuracy.
Enhanced Predictive Accuracy
Improved Data Processing: GPUs can process complex biological data at much higher speeds compared to CPUs. Studies have shown that GPU-enabled predictive models can achieve more accuracy in identifying potential drug candidates. This improvement is crucial for reducing false positives and negatives, thus paving the way for more reliable and effective drug development pipelines.
Sophisticated Algorithms and Simulations: The parallel processing power of GPUs allows for the execution of advanced algorithms and simulations that were previously computationally prohibitive. For instance, molecular dynamics simulations and quantum chemistry calculations, essential for understanding drug interactions at the atomic level, can now be performed more comprehensively. Some configurations have shown up to a reduction in the time required for these complex simulations.
Case Studies and Practical Applications
Industry Implementations
Pfizer’s Genome Sequencing Initiatives: Pfizer has integrated GPU servers into its genome sequencing initiatives. This integration has led to an increase in the accuracy of identifying genetic targets for new drugs, significantly streamlining their research processes and accelerating the timeline for drug discovery.
Novartis’ Drug Screening Program: Novartis employs GPUs in its in silico drug screening programs. Utilizing GPU servers, the company has witnessed an improvement in the accuracy of its predictive models, allowing for the identification of promising compounds with greater confidence. This advancement has facilitated more efficient and cost-effective early-stage drug development.
Operational Benefits
Accelerated Research Timelines: Enhanced predictive accuracy with GPU servers accelerates research timelines. GPU-enabled models can pinpoint promising drug candidates faster, pushing them through the development pipeline more swiftly. This speed is particularly critical when addressing urgent health crises, where rapid drug development can save lives.
Cost Efficiency: More accurate predictive models reduce the likelihood of late-stage failures, which are not only costly but also time-consuming. By minimizing the number of false leads, GPUs help in conserving resources, thereby lowering overall drug development costs. Some estimates suggest potential cost savings thanks to more efficient computational processes.
Challenges and Future Prospects
Integration Challenges
Despite the substantial benefits, integrating GPU servers into existing research infrastructures can be challenging. Compatibility with legacy systems, the need for specialized expertise, and the associated costs of upgrading hardware are significant hurdles. However, the long-term gains in efficiency and accuracy make the investment worthwhile.
Technological Advancements
Looking ahead, the evolution of GPU technology promises even greater enhancements in drug development. Emerging innovations such as tensor processing units (TPUs) and advancements in artificial intelligence (AI) are likely to offer unprecedented computational power. These developments will further refine predictive models, making them more robust and reliable.
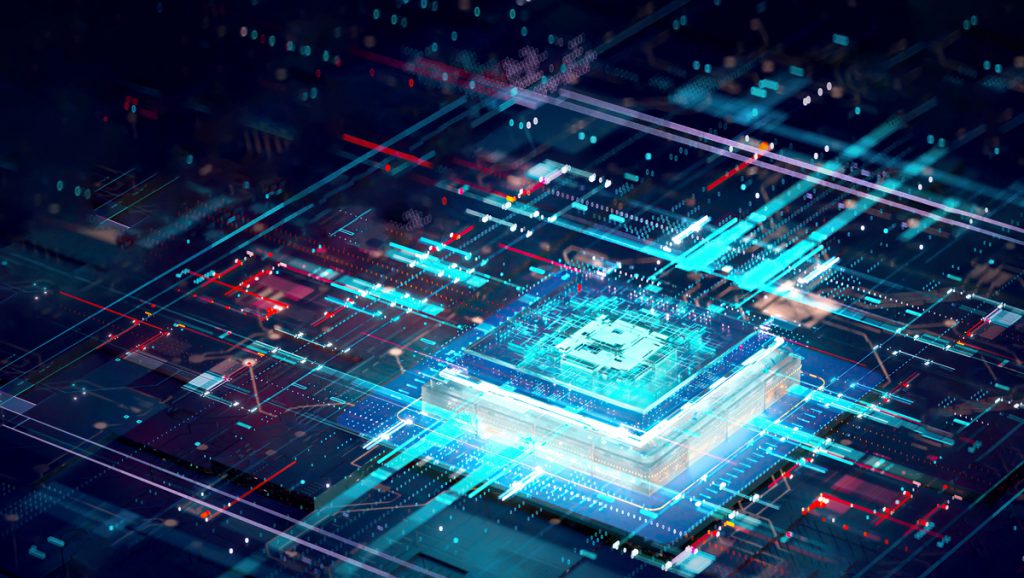
Conclusion
The use of GPU servers in drug development signifies a monumental shift in how predictive models are generated and utilized. Achieving an improvement in predictive accuracy, GPUs offer a powerful solution for managing the complexities of biological data and enhancing drug discovery processes. As stakeholders in the pharmaceutical industry continue to embrace these technological advancements, the future of drug development looks poised for rapid, cost-effective, and highly accurate innovations. The integration of GPU servers is not just a technological upgrade but a strategic necessity for advancing global health.