In the rapidly evolving landscape of artificial intelligence (AI) and machine learning (ML), efficient research productivity hinges on the strategic implementation of scalable FMOps-driven data management and AI workflow automation. By leveraging these advanced techniques, stakeholders can not only streamline operations but also drive groundbreaking innovations. This article delves into the nuances of these methodologies, providing insights and actionable recommendations for maximizing research productivity.
Understanding FMOps and Its Importance
Foundation Model Operations (FMOps) are pivotal in managing and deploying large-scale AI systems, particularly those involving generative AI models. Unlike traditional MLOps, which focus on deploying individual ML models, FMOps orchestrate entire AI ecosystems, offering a unified approach to handle complex AI workflows. This comprehensive strategy ensures scalability, risk reduction, and consistent model performance across various applications.
Benefits of FMOps
Scalability: FMOps support the handling of vast datasets and intricate ML models, facilitating seamless scaling without compromising performance.
Efficiency: Automation and standardized processes expedite model development and deployment, significantly reducing time-to-market.
Quality Assurance: Continuous monitoring and feedback loops lead to the creation of high-quality models, ensuring robust operational performance.
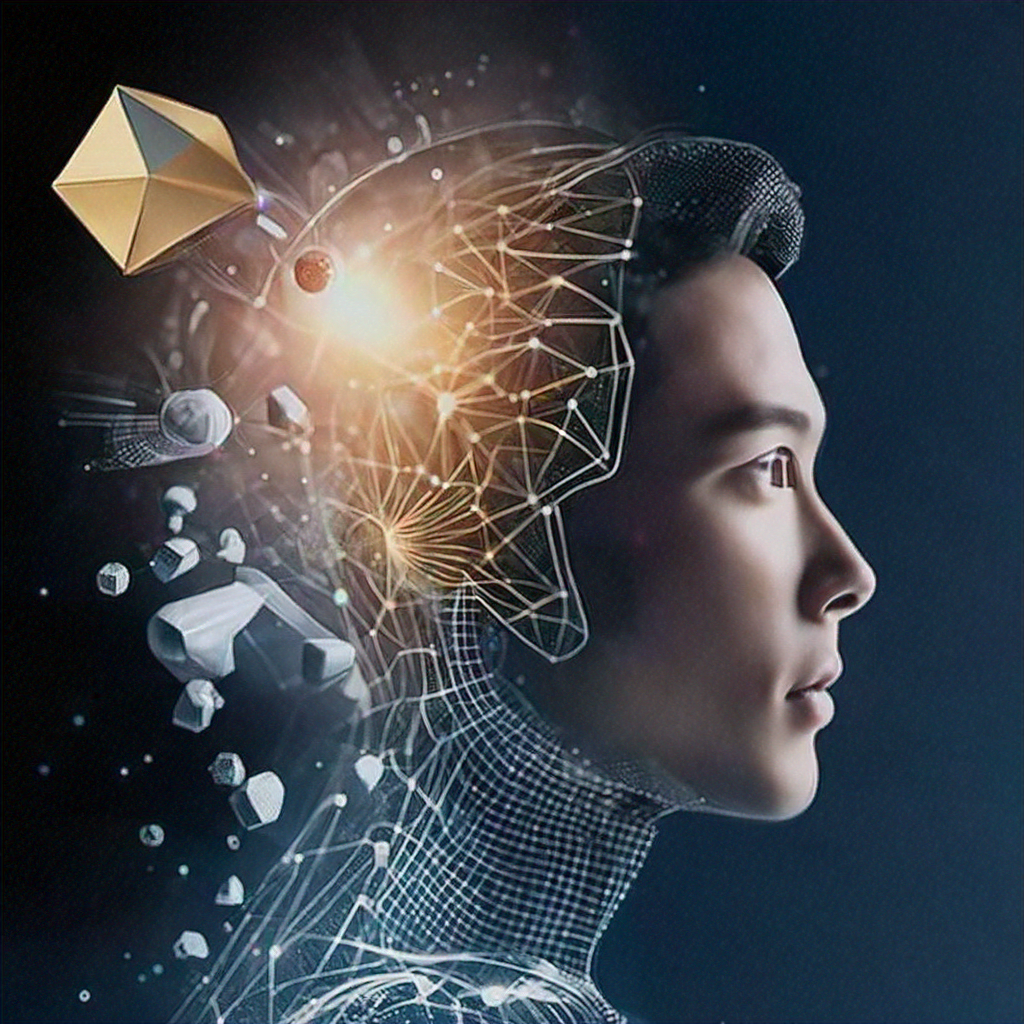
Implementing Scalable FMOps-driven Data Management
Organizations must adopt scalable data management practices to fully harness the potential of FMOps. This involves creating a robust infrastructure that can support extensive data operations, from ingestion to processing and storage.
Key Components of FMOps-driven Data Management
Data Lake and Data Mesh Architecture: These architectures allow for efficient data storage and accessibility, enabling data engineers to curate and catalog data from multiple sources.
ETL Pipelines: Building robust Extract, Transform, Load (ETL) pipelines is crucial for preparing historical data and ensuring data quality, which is foundational for ML use cases. Companies with advanced data practices saw a 270% increase in utilization between 2015 and 2019 (Source: ExplodingTopics)
Data Governance: Implementing strict data governance policies ensures compliance with regulatory requirements and maintains data integrity.
Enhancing Research with Scalable Data Management
Scalable data management directly impacts research productivity by providing researchers with access to comprehensive, high-quality datasets, facilitating more accurate and reliable insights. This allows data scientists to focus on innovative research rather than data wrangling, thus accelerating the pace of discovery.
AI Workflow Automation: A Productivity Catalyst
AI workflow automation significantly enhances research efficacy by automating repetitive tasks, allowing researchers to concentrate on high-value activities.
Core Elements of AI Workflow Automation
Automation of Repetitive Tasks: AI can automate mundane tasks such as data entry, report generation, and basic data analysis, freeing up valuable time for researchers to engage in more complex problem-solving.
Enhanced Collaboration: Automation tools facilitate better communication and collaboration between data scientists, ML engineers, and business stakeholders, ensuring a cohesive approach to research projects.
Continuous Integration/Continuous Deployment (CI/CD): Implementing CI/CD pipelines in AI workflows ensures that models are continuously tested and deployed, enhancing reliability and reducing errors.
Benefits of AI Workflow Automation
Increased Efficiency: Automation leads to substantial time and cost savings, allowing researchers to deliver results faster.
Improved Accuracy: By reducing human errors, AI workflow automation ensures more precise outcomes, which are critical for research validity.
Maximizing Research Productivity: A Strategic Approach
Combining scalable FMOps-driven data management with AI workflow automation creates a powerhouse for research productivity. Here are strategic steps stakeholders can take to maximize research outcomes:
Invest in Robust Infrastructure: Establish a scalable data management system that can handle large volumes of data efficiently. This includes setting up data lakes, ETL pipelines, and robust data governance frameworks.
Enhance Collaboration Through Automation: Use AI tools to automate workflow processes, facilitating seamless collaboration among research teams and ensuring timely and precise outcomes.
Continuous Training and Monitoring: Implement continuous training and monitoring of AI models to ensure they evolve with the latest data and research findings, maintaining their relevance and accuracy.
Adopt a Holistic Approach: Integrate FMOps and AI automation across all research stages, from data collection and preprocessing to model deployment and governance, ensuring a streamlined and cohesive research process.
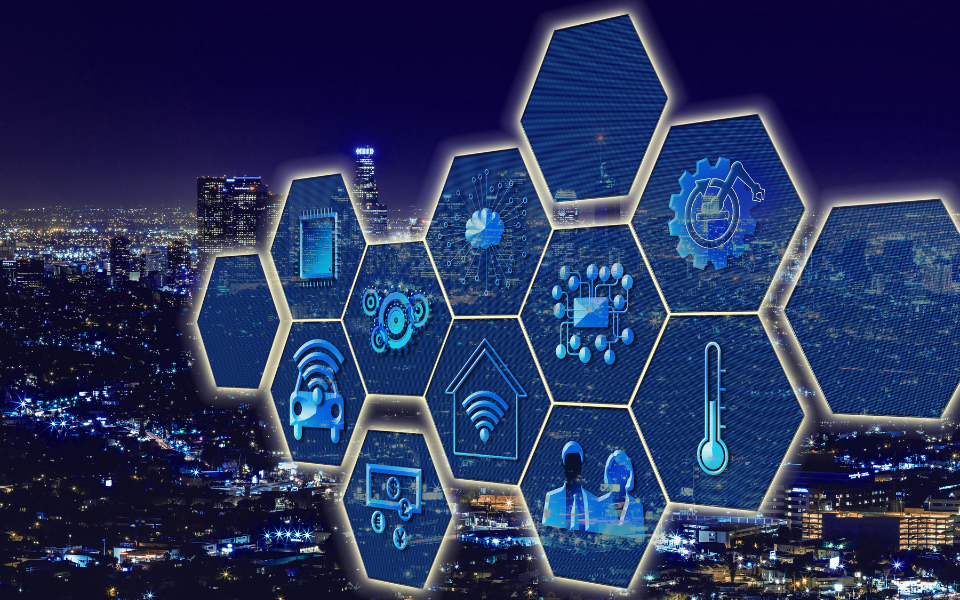
Conclusion
The integration of scalable FMOps-driven data management and AI workflow automation stands as a transformative approach to enhancing research productivity. By adopting these practices, stakeholders can foster innovation, drive efficiency, and achieve groundbreaking discoveries. As the landscape of AI continues to evolve, staying ahead through strategic implementation of these methodologies will be crucial for sustained research excellence.