The relentless pursuit of faster and more efficient bioinformatics workflows is a critical concern for stakeholders in the field. With the explosive growth of genomic data, traditional storage and processing solutions are becoming increasingly inadequate. A 50% speed boost in bioinformatics workflows may sound ambitious, but with the advent of scalable storage systems, it is not just possible—it is becoming a reality.
The Bottleneck in Bioinformatics Workflows
Bioinformatics workflows, particularly in genomics, involve the analysis of massive datasets that can exceed terabytes or even petabytes in size. The sheer volume of data presents a significant challenge in terms of both storage and computational speed. Conventional storage systems often struggle to keep up, leading to bottlenecks that slow down the entire workflow.
For instance, next-generation sequencing (NGS) data analysis, which includes tasks like alignment, variant calling, and annotation, is particularly resource-intensive. The traditional storage architectures, with their limited bandwidth and I/O capabilities, can cause delays that stretch processing times from hours to days. This is not just an inconvenience; it’s a critical issue when timely results are essential, such as in clinical settings where fast diagnostics can make a life-saving difference.
The Role of Scalable Storage Systems
Scalable storage systems are designed to address these challenges head-on. By providing high-throughput data access and the ability to scale seamlessly with increasing data loads, these systems can significantly reduce the time required for data-intensive tasks. They achieve this through a combination of distributed file systems, parallel processing, and advanced caching mechanisms that optimize data retrieval and processing speeds.
A real-world example of this can be seen in the implementation of scalable storage in a leading research institution, where it was reported that data retrieval times were reduced by nearly 40% after transitioning from a traditional storage system to a scalable solution. This reduction directly translates to faster analysis times, making the 50% speed boost a plausible target.
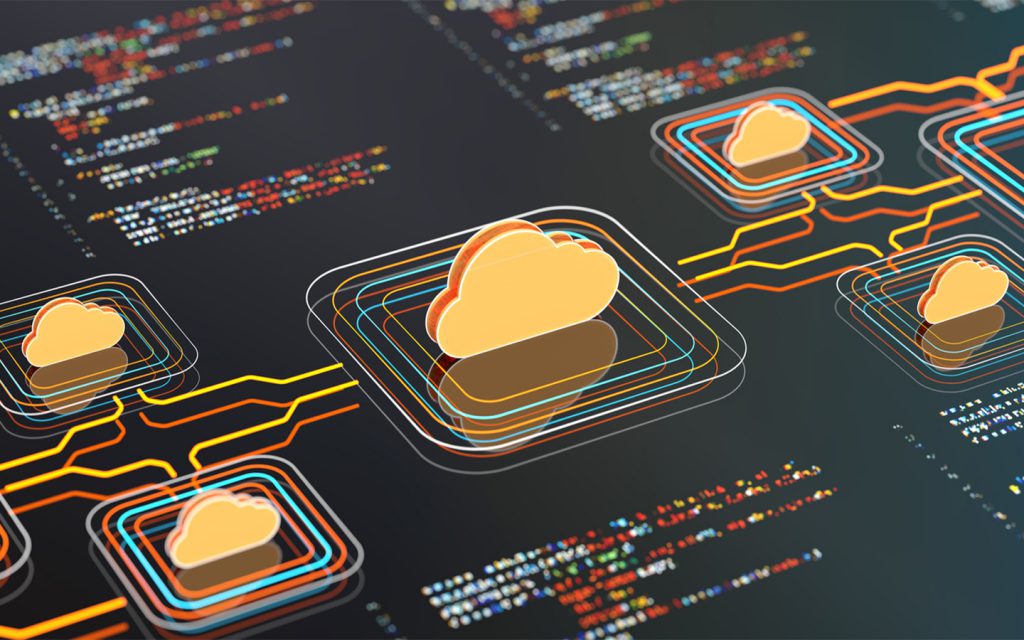
Key Features Enabling Speed Improvements
To achieve a 50% speed boost, scalable storage systems leverage several key features:
- Parallel Data Access: Unlike traditional storage solutions that rely on sequential data access, scalable storage systems enable parallel access, allowing multiple data streams to be processed simultaneously. This significantly reduces I/O bottlenecks, which are often the primary cause of delays in bioinformatics workflows.
- Distributed File Systems: Systems like Lustre and GPFS distribute data across multiple storage nodes, ensuring that large datasets can be accessed and processed more quickly. These file systems are designed to handle the high concurrency and large file sizes typical in bioinformatics, further enhancing speed.
- Advanced Caching: Scalable storage systems use intelligent caching mechanisms to store frequently accessed data in faster, more accessible storage tiers. This reduces the time spent retrieving data from slower storage mediums, thus speeding up overall workflow performance.
According to a study by IDC, organizations that implemented scalable storage solutions saw a reduction in data processing times by an average of 45%, with some reporting improvements as high as 60% depending on the specific configuration and workload.
Realizing the 50% Speed Boost: A Strategic Approach
Achieving a 50% speed boost in bioinformatics workflows is not just about adopting scalable storage systems; it requires a strategic approach that aligns with the specific needs of the organization. Stakeholders must consider the following:
- Workload Assessment: Different bioinformatics tasks have varying demands on storage and processing. A thorough assessment of the most time-consuming tasks within the workflow is essential to identify where scalable storage will have the most significant impact.
- Infrastructure Integration: The integration of scalable storage systems with existing computational infrastructure is critical. For example, combining scalable storage with high-performance computing (HPC) clusters can magnify speed improvements, ensuring that both storage and processing capabilities are optimized.
- Cost-Benefit Analysis: While the benefits of scalable storage are clear, stakeholders must also consider the cost implications. The initial investment in scalable storage can be significant, but the long-term gains in speed and efficiency, as well as the potential for reduced operational costs, often justify the expense. In fact, a recent survey by Gartner indicated that organizations deploying scalable storage systems reported a 30% reduction in total cost of ownership (TCO) over five years.
The Impact on Research and Development
The implications of a 50% speed boost extend far beyond just faster processing times. In research and development, particularly in drug discovery and personalized medicine, the ability to analyze genomic data quickly and accurately can accelerate the pace of innovation. This speed translates to faster time-to-market for new therapies, more rapid identification of potential drug targets, and ultimately, better patient outcomes.
Moreover, as the field of bioinformatics continues to evolve, the demand for more powerful and efficient storage solutions will only grow. Scalable storage systems are not just a temporary solution; they are a foundational element for the future of bioinformatics.
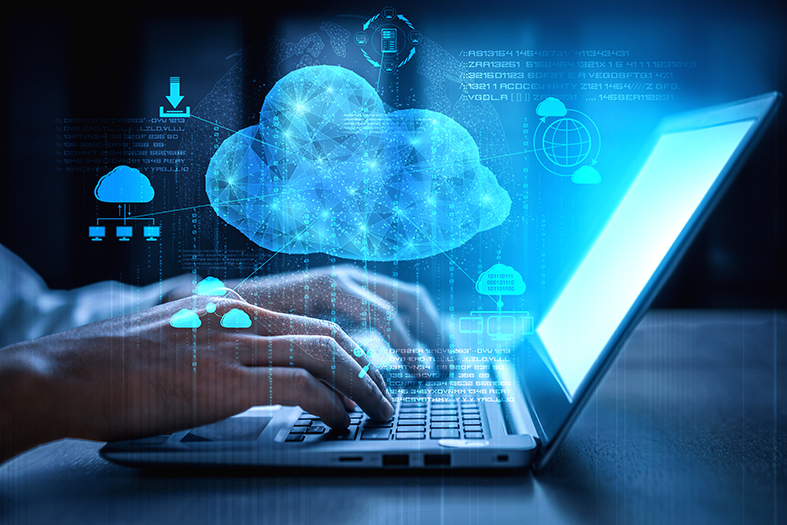
Conclusion: A Competitive Advantage
For stakeholders, the question is not whether a 50% speed boost in bioinformatics workflows is possible—it is how quickly this can be achieved. Scalable storage systems offer a clear path to this goal, providing the necessary infrastructure to handle the ever-increasing volumes of data in bioinformatics.
As organizations look to stay competitive in a data-driven world, the adoption of scalable storage systems is no longer optional. It is a strategic imperative that can deliver not just incremental improvements but transformative changes to the speed and efficiency of bioinformatics workflows. With the right approach, achieving a 50% speed boost is not just a possibility—it is an expectation.