Artificial intelligence (AI) and High Performance Storage solutions (HPS) are transforming the financial industry, enabling finance professionals to unlock new insights, mitigate risks, and capitalize on opportunities in an increasingly dynamic and competitive market landscape. Among the various AI technologies, generative AI is one of the most promising and innovative ones, as it can create novel and realistic data, content, and models based on existing data and knowledge.
Generative AI can be applied to various domains and tasks in finance, such as data augmentation, fraud detection, risk management, portfolio optimization, financial forecasting, customer segmentation, personalized recommendation, and more. However, the adoption and implementation of generative AI in finance is not without challenges, such as data quality, model reliability, ethical issues, and regulatory compliance.
Therefore, it is essential to democratize generative AI, which means making it accessible, affordable, and easy to use for finance customers, regardless of their technical expertise, budget, or location. Democratized generative AI can empower finance customers to create and test new financial models and strategies, enhance their decision making, and improve their financial outcomes. In this article, we will explore some of the benefits and use cases of democratized generative AI for finance customers, as well as some of the best practices and considerations for its successful deployment.
Use Cases of Democratized Generative AI for Finance Customers
Democratized generative AI can be applied to various use cases and scenarios in finance, such as:
- Fraud detection: Generative AI can help finance customers detect and prevent fraudulent activities, such as identity theft, money laundering, credit card fraud, etc. By generating synthetic fraud cases based on real data, generative AI can help finance customers train and test their fraud detection models, and improve their accuracy and robustness. Moreover, generative AI can help finance customers identify and flag anomalous and suspicious transactions and behaviors, and alert them in real time.
- Risk management: Generative AI can help finance customers manage and mitigate various types of risks, such as market risk, credit risk, operational risk, etc. By generating synthetic risk scenarios based on real data, generative AI can help finance customers assess and quantify their risk exposure, and evaluate their risk appetite and tolerance. Furthermore, generative AI can help finance customers optimize their risk mitigation strategies, and allocate their resources and capital efficiently.
- Portfolio optimization: Generative AI can help finance customers optimize their portfolio performance, and achieve their financial objectives and constraints. By generating synthetic portfolio returns and risks based on real data, generative AI can help finance customers simulate and compare different portfolio compositions and allocations, and select the optimal one. Additionally, generative AI can help finance customers monitor and adjust their portfolio dynamically, and respond to changing market conditions and customer preferences.
- Financial forecasting: Generative AI can help finance customers forecast and predict various financial variables and indicators, such as stock prices, exchange rates, interest rates, inflation rates, etc. By generating synthetic financial time series based on real data, generative AI can help finance customers capture and model the complex and nonlinear relationships and trends of financial data, and generate realistic and diverse forecasts. Moreover, generative AI can help finance customers perform what-if analysis and scenario planning, and prepare for different outcomes and contingencies.
- Customer segmentation: Generative AI can help finance customers segment and target their customers based on various attributes and criteria, such as demographics, psychographics, behavior, etc. By generating synthetic customer profiles based on real data, generative AI can help finance customers create and refine their customer segments, and understand their characteristics and needs. Furthermore, generative AI can help finance customers personalize and customize their marketing and communication strategies, and deliver the right message to the right customer at the right time.
- Personalized recommendation: Generative AI can help finance customers provide personalized and relevant recommendations and advice to their customers, and enhance their customer experience and engagement. By generating synthetic customer feedback and ratings based on real data, generative AI can help finance customers learn and infer their customer preferences and interests, and recommend the most suitable financial products and services. Additionally, generative AI can help finance customers nudge and guide their customers to make better financial decisions, and achieve their financial goals and aspirations.
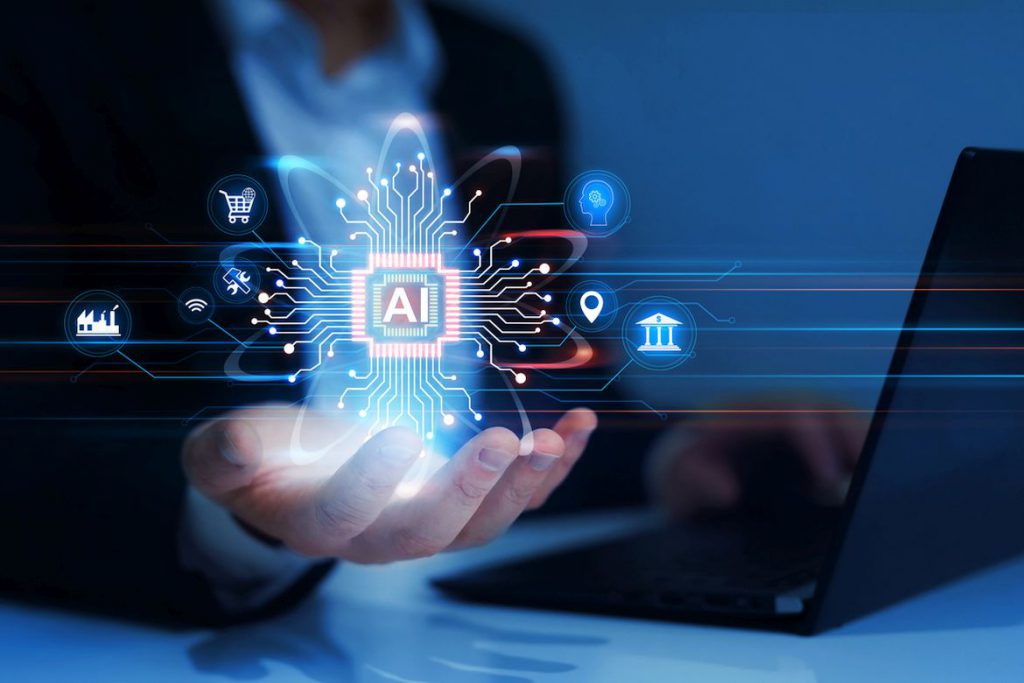
Best Practices and Considerations for Democratized Generative AI for Finance Customers
Democratized generative AI can offer many opportunities and advantages for finance customers, but it also poses many challenges and risks, such as:
- Data quality: Generative AI relies on the quality and quantity of the data that it is trained and tested on, and the data that it generates. Therefore, finance customers need to ensure that their data is accurate, complete, consistent, and representative of the real world, and that their generative AI models are validated and verified for their reliability and validity. Moreover, finance customers need to monitor and evaluate the quality and diversity of the data that generative AI produces, and avoid any biases, errors, or anomalies that may affect their analysis and modeling.
- Ethical issues: Generative AI raises many ethical issues and concerns, such as privacy, security, accountability, transparency, and fairness. Therefore, finance customers need to respect and protect the privacy and security of their data and their customers’ data, and comply with the relevant laws and regulations. Moreover, finance customers need to ensure that their generative AI models are accountable and transparent for their decisions and actions, and that they are fair and unbiased for their outcomes and impacts.
- Regulatory compliance: Generative AI is subject to various regulatory and legal frameworks and standards, such as GDPR, CCPA, FINRA, etc. Therefore, finance customers need to be aware and informed of the regulatory and legal requirements and implications of using and deploying generative AI in finance, and adhere to the best practices and guidelines. Moreover, finance customers need to communicate and collaborate with the regulators and stakeholders, and demonstrate and explain their generative AI models and results.
To successfully implement and leverage democratized generative AI for finance, finance customers need to adopt a holistic and strategic approach, and consider the following steps and factors:
- Define the problem and the objective: Finance customers need to clearly define the problem that they want to solve or the objective that they want to achieve with generative AI, and identify the relevant data and metrics that they need to collect and measure.
- Select the appropriate generative AI model and tool: Finance customers need to select the most suitable generative AI model and tool for their problem and objective, and evaluate their performance and feasibility. Some of the popular and widely used generative AI models and tools are Generative Adversarial Networks (GANs), Variational Autoencoders (VAEs), Transformers, etc.
- Train and test the generative AI model and tool: Finance customers need to train and test their generative AI model and tool on their data, and optimize their parameters and hyperparameters. They also need to validate and verify their generative AI model and tool for their accuracy and robustness, and compare and benchmark their results with other models and tools.
- Deploy and monitor the generative AI model and tool: Finance customers need to deploy and monitor their generative AI model and tool in their production environment, and integrate them with their existing systems and processes. They also need to update and maintain their generative AI model and tool regularly, and respond to any feedback or issues that may arise.
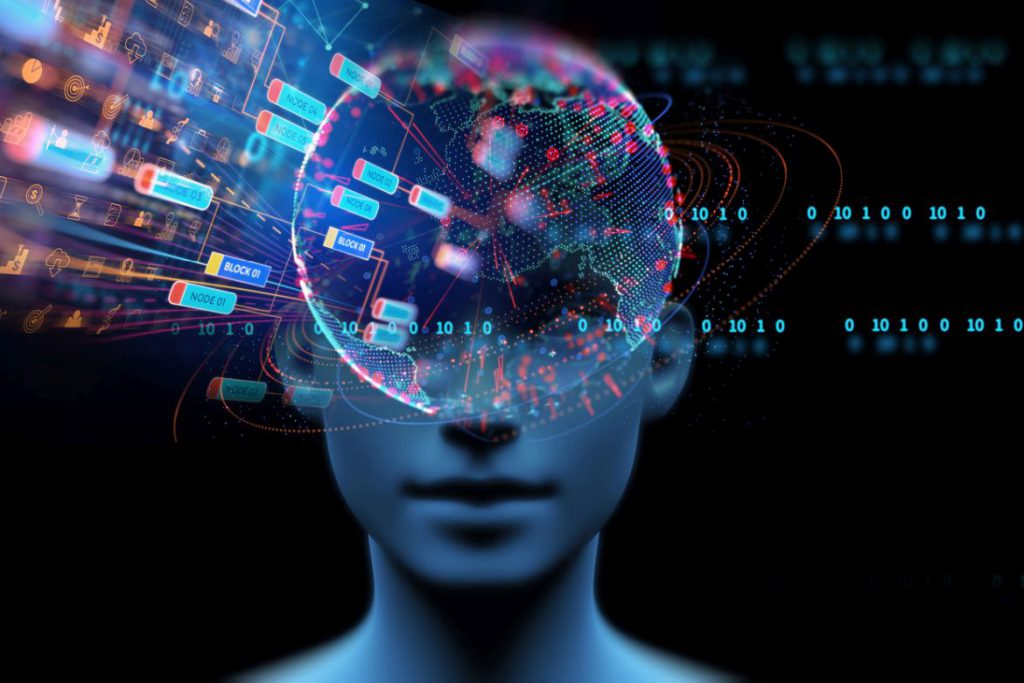
Conclusion
Democratized generative AI is a powerful and innovative technology that can enable finance customers to create and test new financial models and strategies, enhance their decision making, and improve their financial outcomes. However, democratized generative AI also entails many challenges and risks, such as data quality, ethical issues, and regulatory compliance. Therefore, finance customers need to adopt a holistic and strategic approach, and follow the best practices and considerations for its successful deployment. By doing so, finance customers can harness the full potential and value of democratized generative AI, and transform the financial industry and society for the better.