As businesses increasingly leverage machine learning (ML) to drive innovation, the significance of integrating machine learning operations (MLOps) within cloud-native environments cannot be overstated. Cloud-native MLOps, which refers to the practice of deploying and managing ML models using cloud services and solutions, is transforming the landscape by offering unparalleled scalability, efficiency, and collaboration. This article delves into the profound impact of cloud-native MLOps on machine learning, outlining its benefits, key components, and future trends.
The Genesis of Cloud-Native MLOps
MLOps, a subset of ML engineering, focuses on streamlining the development, deployment, and monitoring of ML models. By advocating for continuous delivery and automation, MLOps bridges the gap between data scientists and operational teams, ensuring that models transition smoothly from development to production. The introduction of cloud-native solutions amplifies this efficiency, enabling businesses to harness the full power of the cloud for ML workflows. According to Gartner’s study on the top trends shaping the future of data science and ML, integrating operations within the cloud is a game changer for organizations looking to drive data-centric initiatives (Source: Gartner).
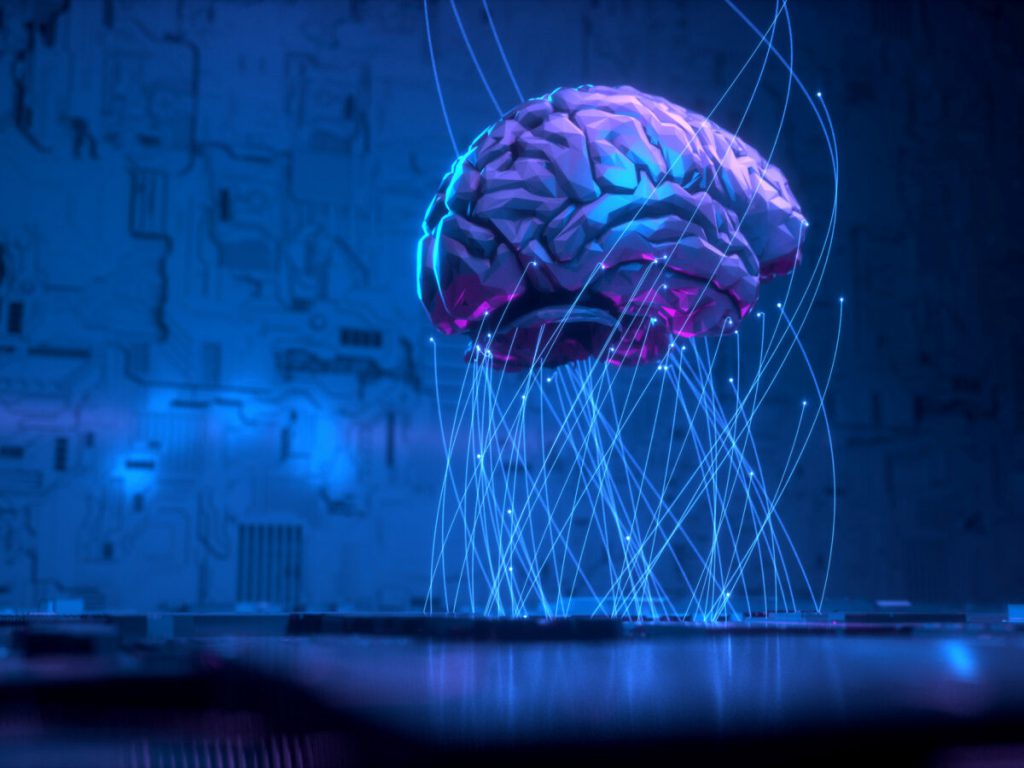
Advantages of Cloud-Native MLOps
1. Scalability and Flexibility
One of the primary advantages of deploying ML models on the cloud is high scalability. Cloud providers offer robust infrastructure that can scale resources as needed. This is particularly beneficial for ML projects, which often require significant computational power. Symphony Solutions reports that businesses can easily test and iterate on models simultaneously, supporting both experimentation and production scaling with ease (Source: Symphony Solutions).
2. Enhanced Collaboration and Efficiency
Cloud-native MLOps fosters collaboration between data scientists, DevOps engineers, and IT teams. By leveraging centralized platforms, teams can work together more effectively, reducing the frequency and severity of issues. Gartner highlights that 50% of new system deployments in 2024 will be based on cohesive cloud data ecosystems, reflecting a shift towards integrated, collaborative environments that enhance model development efficiency (Source: Gartner).
3. Cost-Effectiveness
Implementing MLOps on cloud platforms also brings cost benefits. Cloud services operate on a pay-as-you-go model, allowing businesses to control expenditures and invest only in the resources they need. MLOps further optimizes this by automating repetitive tasks and version control, thus saving time and reducing developmental costs, as noted by InfraCloud (Source: InfraCloud).
Key Components of Cloud-Native MLOps
1. Continuous Integration and Continuous Delivery (CI/CD)
CI/CD pipelines are at the heart of cloud-native MLOps, ensuring the automatic integration, testing, and deployment of ML models. These pipelines facilitate rapid iterations and quicker deployments, maintaining high model accuracy and reliability over time. Google Cloud emphasizes that automation in CI/CD pipelines improves overall workflow, making ML model management more seamless (Source: Google Cloud).
2. Experiment Tracking and Model Monitoring
Cloud-native MLOps platforms provide advanced tools for tracking experiments and monitoring model performance. Neptune.ai, for instance, offers solutions that support scaling and feature enhancements, enabling teams to monitor training and compare experiments effectively. This continuous monitoring is crucial for identifying performance degradation and ensuring models meet business needs (Source: Neptune.ai).
3. Data Management and Compliance
Effective data management is central to MLOps, especially within cloud environments. Gartner’s focus on data-centric AI highlights the critical need for managing data accessibility, privacy, and security. Techniques such as synthetic data generation and AI-specific data management are rising to address these challenges, making data more usable and secure for ML applications (Source: Gartner).
Future Trends in Cloud-Native MLOps
1. Edge AI Integration
The demand for Edge AI, which processes data at the point of creation, is increasing. Gartner predicts that by 2025, over 55% of data analysis by deep neural networks will occur at the edge, up from less than 10% in 2021. This trend underlines the shift towards real-time insights and stringent data privacy requirements, driving the need for cloud-native MLOps solutions that can integrate with edge environments (Source: Gartner).
2. Responsible AI Practices
As AI technologies become more pervasive, adopting responsible AI practices is paramount. Responsible AI encompasses ethical considerations such as risk management, transparency, and accountability. By 2025, Gartner anticipates that the concentration of pre-trained AI models among a small percentage of vendors will make responsible AI a societal concern, necessitating robust compliance and ethical frameworks within MLOps platforms (Source: Gartner).
3. Accelerated Investment in AI and ML
Investment in AI continues to surge, driven by the capability to solve complex business problems and foster innovation. More than $10 billion is expected to be invested in AI startups by the end of 2026, with substantial interest in generative AI technologies. Gartner’s report shows that 45% of executives have increased their AI investments, influenced by the hype around technologies such as ChatGPT (Source: Gartner).
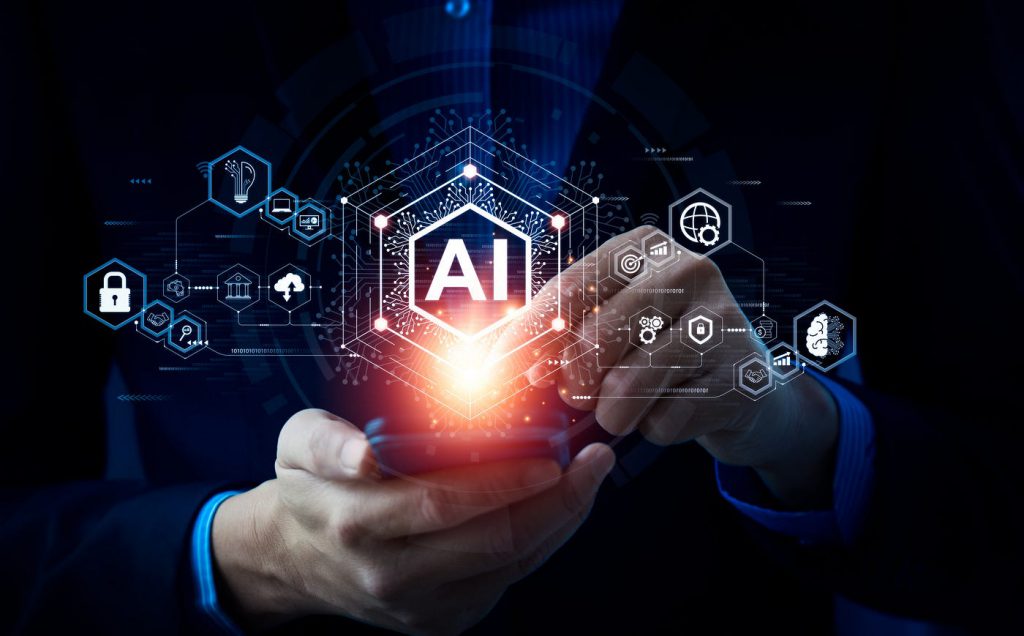
Conclusion
Cloud-native MLOps is reshaping the future of machine learning by providing scalable, efficient, and collaborative solutions that span the entire ML lifecycle. With significant advantages, including scalability, enhanced collaboration, and cost-effectiveness, businesses are better equipped to develop, deploy, and iterate on ML models. Looking forward, trends such as Edge AI, responsible AI practices, and accelerated AI investments will further solidify the role of cloud-native MLOps in driving innovation and growth across industries. By leveraging the cloud’s capabilities and integrating MLOps best practices, organizations can ensure that their machine learning initiatives are both effective and sustainable, paving the way for a data-driven future.