Introduction
In this highly competitive financial landscape, core banking platforms are essential for providing uninterrupted services such as account management, transaction processing, and loan disbursement. Stakeholders expect nearly flawless service levels to maintain customer trust, comply with regulatory requirements, and prevent revenue loss. Even minor delays or unexpected outages can result in multi-million-dollar penalties and damage to a company’s reputation.
The global AIOps market is projected to reach $99.07 billion by 2025, growing at a compound annual growth rate (CAGR) of over 38%. This growth is driven by the increasing demand for real-time operational intelligence in mission-critical areas such as core banking. This rapid expansion emphasizes the urgent need for financial institutions to integrate AIOps into their IT strategies. (Source: ResearchNester)
To address these challenges, real-time Service Level Agreement (SLA) assurance—enhanced by artificial intelligence for IT operations (AIOps)—has emerged as a transformative strategy. This approach helps ensure operational continuity and unlocks new levels of efficiency.
The Stakes of SLA Breaches in Core Banking
- Regulatory Exposure: Financial regulators across key markets (e.g., PSD2 in Europe, RBI guidelines in India, OCC directives in the U.S.) impose strict uptime and latency thresholds. A single SLA breach can trigger fines, mandated audits, or even forced remediation plans.
- Revenue & Customer Churn: According to industry analyses, a one-hour system outage in a mid-sized bank can translate to direct transaction losses, with a secondary cost in attrition when customers migrate to more reliable competitors.
- Reputational Impact: Brand value in banking is tightly coupled with reliability. Social media amplifies even isolated service disruptions into global news cycles, eroding stakeholder confidence.
Limitations of Traditional Monitoring Approaches
- Reactive Alerting: Threshold-based alerts trigger only after performance metrics cross predefined limits, often resulting in “noisy” alert storms that desensitize IT teams.
- Data Silos: Disparate log, metric, and trace repositories hinder end-to-end visibility. By the time teams piece together events, the root cause may have shifted.
- Manual Remediation: Escalation chains and manual script execution introduce latency and human error, undermining SLAs rather than protecting them.
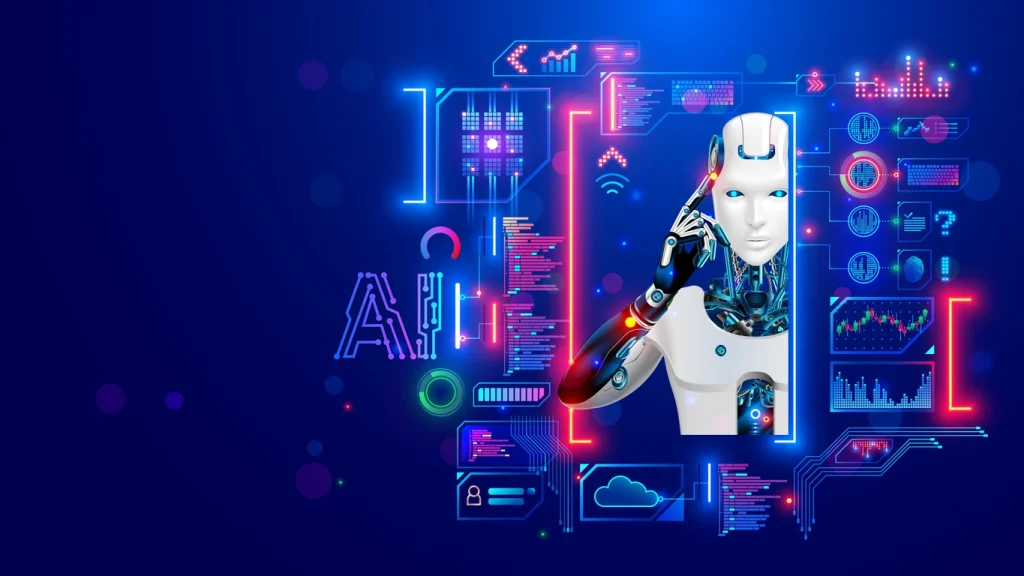
AIOps-Driven Real-Time SLA Assurance
AIOps unifies machine learning, automation, and big-data processing to convert raw telemetry into predictive insights and automated actions. In core banking environments, this paradigm shift enables:
1. Anomaly Detection at Scale
- Adaptive Baselines: ML models continuously learn transaction volume patterns, infrastructure load curves, and end-user behavior. Sudden deviations—such as a spike in database response times—trigger immediate investigation tickets before SLAs are impacted.
- Noise Reduction: By correlating alerts across multiple telemetry streams, AIOps platforms suppress redundant notifications, surfacing only the highest-severity incidents to engineering and operations teams.
2. Predictive Remediation
- Forecasting Degradation: Time-series forecasting algorithms project potential breaches in CPU, memory, or network thresholds hours before they occur. This advance warning allows orchestration engines to spin up capacity or reconfigure workloads proactively.
- Automated Runbooks: Pre-tested, governance-approved remediation scripts execute without human intervention—redeploying microservices, clearing cache backlogs, or rerouting traffic to healthy nodes within seconds.
3. Unified Observability & Contextual Root-Cause Analysis
- Integrated Data Fabric: Telemetry from mainframes, UNIX/Linux servers, container clusters, and messaging buses is ingested into a centralized platform. Graph-based dependency maps contextualize service chains—so when a failed middleware component threatens the payments queue, the system identifies the exact failure path in real time.
- Causal Inference: Beyond correlation, causal ML models discern whether a database lock contention is driving API latencies or vice versa, cutting mean time to resolution (MTTR).
Quantifiable Business Impact
- Reduced Breach Penalties: Institutions report fewer SLA violations within six months of deploying AIOps, translating into millions of dollars saved in regulatory fines.
- Lower Operational Overhead: Automated remediation diminishes repetitive toil for NOC and DevOps teams, reallocating staff effort toward innovation projects.
- Improved Customer Experience: Real-time incident prevention results fewer customer complaints and higher net promoter scores (NPS).
Implementation Considerations
- Data Governance & Privacy
- Financial data is highly regulated. Ensure telemetry pipelines comply with data sovereignty laws, masking or anonymizing personally identifiable information (PII) where required.
- Model Validation & Testing
- Machine learning in production demands ongoing validation. Establish feedback loops to retrain models with new performance profiles and avoid “drift.”
- Runbook Certification
- Automations must undergo rigorous testing in pre-production environments. Incorporate compliance checkpoints to prevent unauthorized actions on critical systems.
- Cross-Functional Collaboration
- Align IT operations, security, compliance, and business units to define SLA parameters, escalation workflows, and remediation policies. AIOps success hinges on clear, shared objectives.
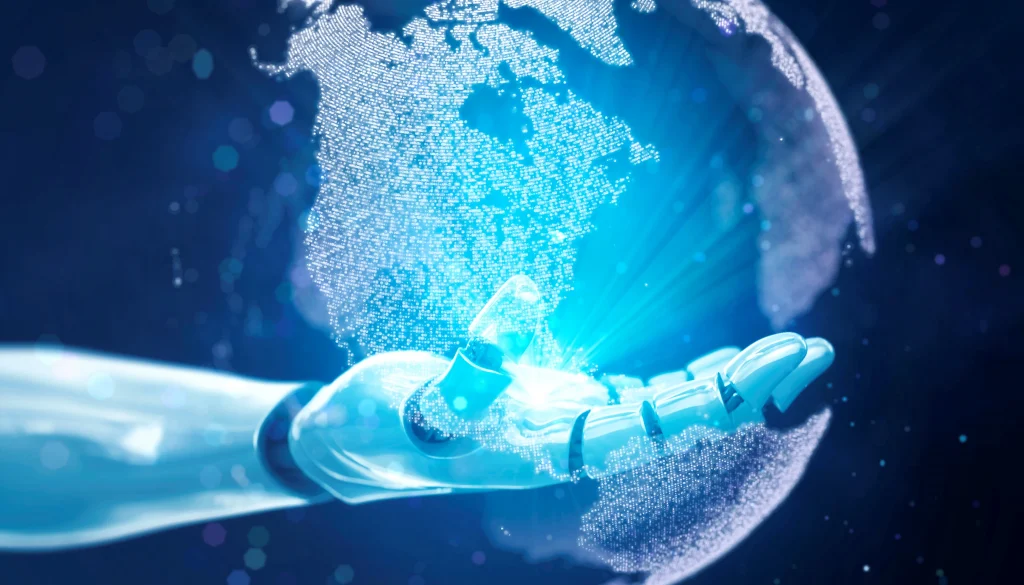
Conclusion
For core banking platforms, real-time SLA assurance is no longer a theoretical advantage—it is a strategic imperative. By harnessing AIOps for predictive insights, automated remediation, and unified observability, financial institutions can proactively defend against service disruptions, optimize resource utilization, and strengthen regulatory compliance. Stakeholders looking to unlock operational efficiency must evaluate AIOps as the cornerstone of their next-generation monitoring strategy, ensuring that SLAs become a competitive differentiator rather than a constant risk.