Introduction
AI research today demands infrastructure that adapts as quickly as ideas flow. Traditional data‑center models, with fixed GPU assignments, create bottlenecks, force lengthy provisioning cycles, and silo domain expertise. Composable GPU workspaces decouple compute, storage, and networking into software‑defined pools, enabling teams to spin up tailored environments on demand. The result is a seamless bridge between data scientists, domain experts, and engineers, fostering cross‑disciplinary breakthroughs without the friction of hardware constraints.
Architecture of Composable GPU Workspaces
Decoupling and Dynamic Allocation
Composable workspaces treat GPUs, NVMe storage, and high‑speed interconnects (e.g., PCIe‑fabric, NVLink‑equivalent fabrics) as modular services. An orchestration layer dynamically assembles these services into virtual clusters within minutes, scaling from a single GPU for rapid prototyping to multi‑GPU arrays for large‑scale training. Secure multi‑tenant virtualization ensures each research group receives guaranteed performance and isolation, so exploratory experiments and production workloads run side by side without contention.
Mission‑Critical Efficiency Gains
By aligning infrastructure spend directly with usage, organizations eliminate idle capacity and slash total cost of ownership. Rather than pre‑purchasing oversized GPU farms, teams consume resources as needed, converting CapEx to OpEx. This fluid model not only reduces waste but also accelerates R&D budgets, reallocating savings toward innovation rather than idle assets.
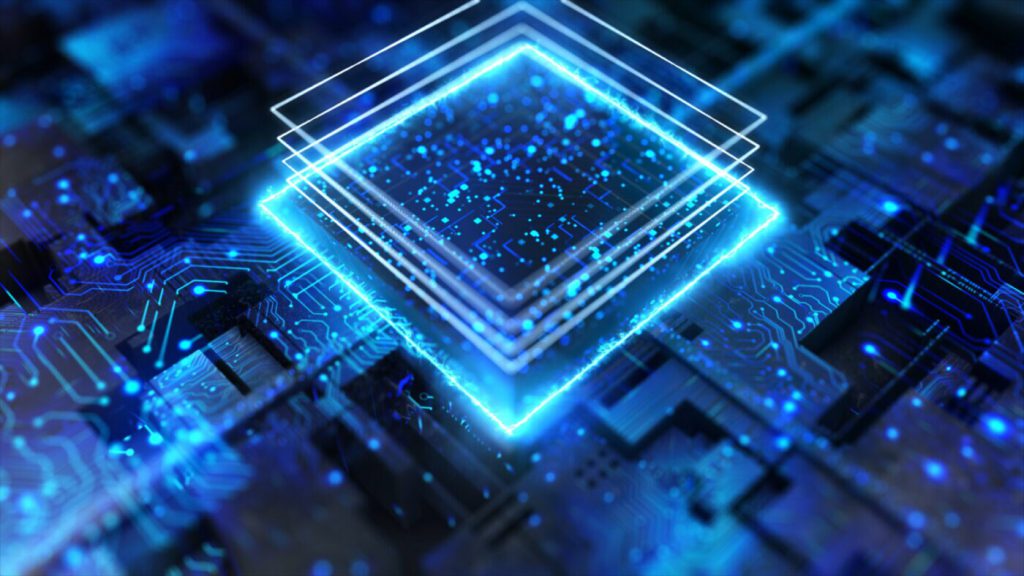
Enabling Cross‑Disciplinary Breakthroughs
Shared Virtual Environments
Uniform, cloud‑like environments guarantee that genomics researchers, materials scientists, and software engineers all see the same libraries, drivers, and datasets. This consistency eradicates “it works on my laptop” debates. Artifacts, such as trained model checkpoints, can be versioned and shared across departments, enabling domain experts to build upon each other’s work without translation overhead.
Real‑World Case Studies
- Drug Discovery Acceleration
A major research university deployed composable GPU nodes to streamline molecular‑simulation workflows. Training cycles shrank from days to hours, letting biochemists iterate on compound libraries in real time.
- Generative Engineering Design
An aerospace consortium ran hundreds of design variants in parallel, compressing concept‑to‑prototype timelines by over a third and uncovering novel lightweight structures.
- Fine‑Grained Multi‑Workload Scheduling
R&D labs using virtual GPU partitions saw dramatic utilization improvements, seamlessly packing small‑scale experiments alongside heavy‑train jobs without performance degradation.
Stakeholder Benefits
Cost Optimization & ROI
By provisioning GPUs only when needed, organizations unlock significant savings. With these savings, CFOs can reallocate budget toward strategic initiatives, fueling additional AI projects rather than covering idle hardware.
Performance & Market Dynamics
GPU hardware has advanced exponentially: a report by Stanford’s Human‑Centered AI group found that GPU performance has increased approximately 7,000× since 2003, with a price‑performance ratio 5,600× greater (Source: Stanford Human‑Centered AI group). These gains mean composable workspaces can harness cutting‑edge accelerators as they emerge, ensuring R&D teams always operate at the performance frontier.
Strategic Adoption Trends
Composable architectures are moving from “emerging” to “mainstream.” Gartner’s 2025 Infrastructure & Operations Roadmap reports that 62% of assessed I&O technologies, among them composable systems, are already in deployment (Source: Gartner I&O Roadmap). This signals that organizations embracing composable GPU workspaces today will be aligned with the industry’s leading edge.
Market Outlook & Imperative
The global GPU market is projected to soar from USD 61.58 billion in 2024 to USD 461.02 billion by 2032, at a CAGR of 28.6% (Source: Fortune Business Insights). As demand for AI accelerators skyrockets, securing flexible, composable access becomes a strategic differentiator, preventing supply‑chain lock‑ins and enabling on‑demand scale.
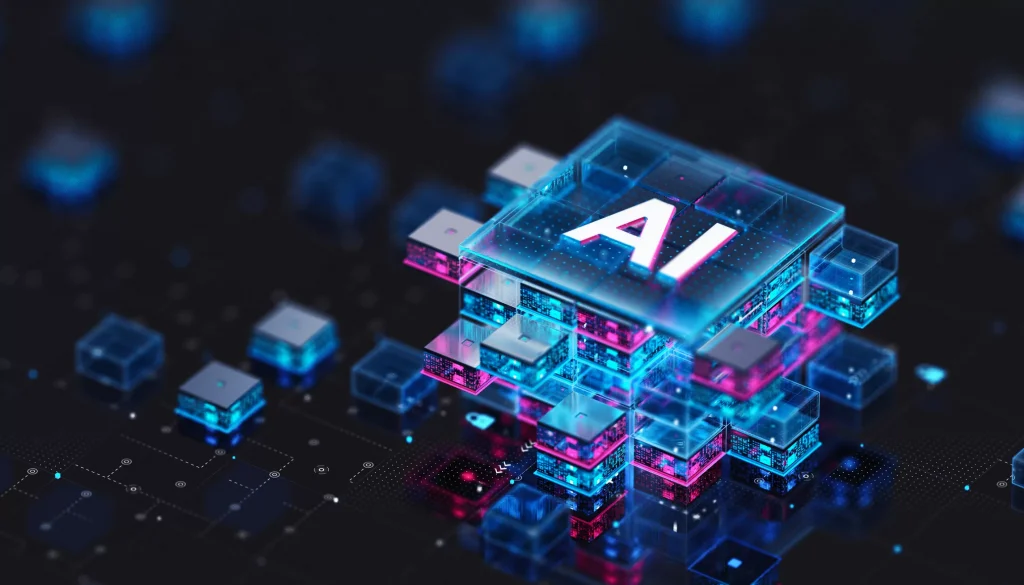
Conclusion
In an era where AI breakthroughs require agility, cost efficiency, and seamless collaboration, composable GPU workspaces deliver a new infrastructure paradigm. Stakeholders gain not only dramatic cost savings and performance gains but also the ability to unite cross‑disciplinary teams in a shared, on‑demand environment. With proven performance improvements, rapid adoption by industry leaders, and a booming GPU market, now is the moment to redefine AI R&D collaboration through composable GPU workspaces, and lock in a decisive competitive advantage.